“Feels like we’ve done years of work in just a few months,” confessed a weary engineer from Chinese automaker Li Auto’s autonomous driving team, reflecting on the intense development journey of the past half-year.
This sentiment captures the essence of Li Auto’s relentless sprint over the past year. CEO Li Xiang’s midnight tests of autonomous features and the subsequent bug-fixing sessions that kept engineers burning the midnight oil have become emblematic of the company’s commitment to innovation. Working seven days a week, often into the early hours, has become the new normal during this closed development phase.
In 2023, the autonomous driving arena in China witnessed a surge in activity, with companies like Huawei, Xpeng Motors, and Li Auto engaging in a fierce battle for market dominance. Despite this, no clear leader has emerged, driving each competitor to push harder while also keeping a close eye on their rivals’ progress.
The competition has expanded nationwide this year, with companies like Huawei boldly declaring their capability to enable autonomous driving on all roads across China. Nio quickly followed suit, rolling out similar features to its user base. Although Xpeng and Li Auto have been more discreet about their timelines, both have initiated small-scale testing of nationwide self-driving capabilities.
However, the entry of Tesla’s Full Self-Driving (FSD) package into the Chinese market has added a new dimension to the competition, leaving competitors scrambling to keep up. While Tesla may have entered the scene later than others, its momentum is undeniable, raising speculation about the potential impact of its offerings.
Amid a fiercely competitive landscape and a price war that has strained even industry leaders like Tesla, autonomous driving remains one of the few variables in the automotive industry’s high-stakes game. How far are we from witnessing its full-scale disruption?
Buzz and skepticism
On March 19, Aito, a subsidiary of Huawei, made waves by announcing the full-scale deployment of advanced autonomous driving features to users. Claiming coverage of 99% of China’s roads, Aito’s initiative concretizes the concept of “nationwide” by specifying the inclusion of 40,000 Chinese towns and villages. Its goal is to have 500,000 vehicles equipped with Huawei’s autonomous driving system on the roads by the end of 2024.
This breakthrough has not only opened new possibilities but also intensified the competitive pressure on other players in the industry. For competitors, falling behind Huawei in this aspect could further dampen their brand visibility and sales prospects.
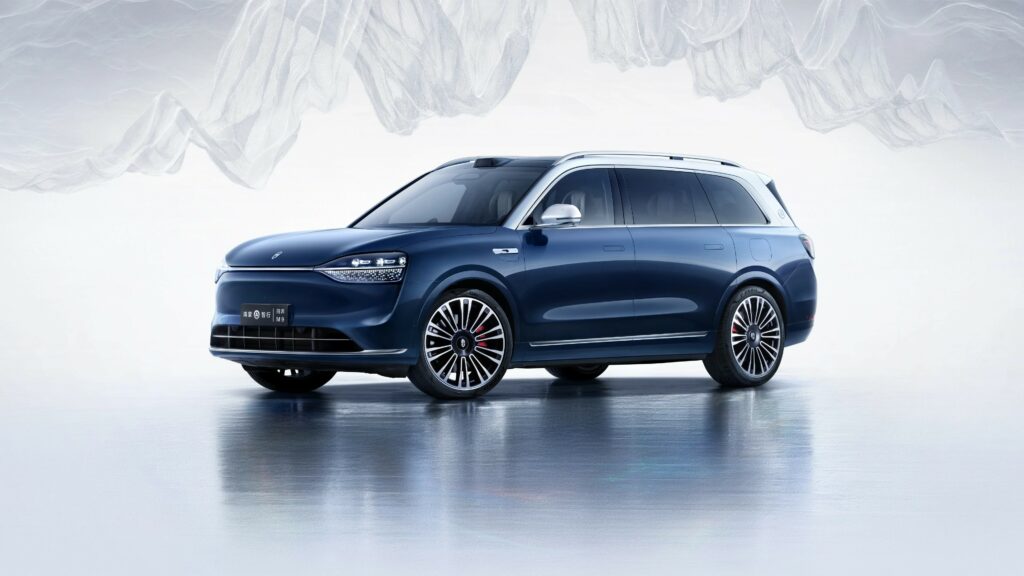
For Nio, facing a year without new car releases, autonomous driving has emerged as one of the few strategies to drive sales. Following in Huawei’s footsteps, on April 30, Nio became the second Chinese automaker to roll out advanced autonomous driving features, extending its coverage to over 200,000 users.
Similarly, Xpeng has consistently positioned autonomous driving as a strategic advantage that cannot be relinquished. Meanwhile, Li Auto is transitioning toward becoming an artificial intelligence technology company and is determined to remain at the forefront of self-driving innovation.
Insights from 36Kr reveal that, despite the use of bold marketing slogans, actual deployments of autonomous driving features by automakers tend to be relatively cautious. Both Huawei and Nio, for instance, require users to pass assessments and tests before enabling autonomous driving in urban areas. Additionally, users need to accumulate a certain amount of autonomous driving mileage on highways before they are allowed to utilize it in city centers.
However, skepticism is rife among industry professionals. For one, definitions of nationwide capabilities appear to vary between automakers—while Huawei emphasizes granular coverage, Nio focuses on road mileage, and Xpeng prioritizes system intervention frequency.
This inconsistency in standards also clouds the intensity of testing. With the vastness of China’s road network, it is nearly impossible for automakers to test every single road before rolling out features to users.
Moreover, achieving nationwide capabilities does not necessarily guarantee usability and functionality. Challenges persist in machine perception, especially at large intersections, where factors like traffic congestion and weather conditions can affect system performance. Evaluating the effectiveness of autonomous driving features requires considering their consistency across various scenarios.
To address these challenges, some technical managers advocate for classifying autonomous driving products into three levels: usable, functional, and user-friendly. While Tesla has achieved functionality and begun to touch upon user-friendliness, domestic industry leaders are still navigating between usability and functionality. The concept of nationwide autonomous driving capabilities merely denotes usability, leaving room for refinement in user experience and other areas.
Ren Shaoqing, head of autonomous driving at Nio, told 36Kr that it will take at least two years to achieve this—the first year will focus on ensuring the robustness of features, followed by another year of continuous optimization for user experience.
While achieving the concept of “nationwide autonomous driving” marks a milestone in the industry, it also signals the beginning of a new phase focused on product experience refinement and prolonged competition. This journey hinges on the continuous implementation of end-to-end and other emerging technologies.
End-to-end: Gimmick or panacea?
Tesla’s FSD V12 has made significant strides in the realm of autonomous driving. Powered by an end-to-end neural network, this version represents the next-generation solution for autonomous driving.
Traditional autonomous driving systems typically comprise multiple independent modules such as perception, prediction, planning, and control. These systems rely heavily on rules coded by engineers to govern how autonomous vehicles operate.
In contrast, end-to-end solutions treat sensor data as input and output vehicle control commands, processing them directly. By handling the entire process, information transfer can occur seamlessly.
Essentially, end-to-end solutions are built to autonomously learn and replicate human driving experiences through extensive data training.
An industry expert told 36Kr that, with end-to-end solutions, autonomous driving systems can exhibit more human-like behaviors, especially in navigating complex urban intersections, leading to smoother processes like traffic light management.
However, Tesla has not disclosed the specific technical implementation of its end-to-end solution, posing a challenge for the industry to ascertain whether it has truly pulled off the feat and abandoned intermediate steps customary in traditional modules.
An industry executive said to 36Kr that domestic end-to-end solutions are unlikely to adopt Tesla’s radical approach and will instead opt for a more moderate strategy.
Since the collective abandonment of high-precision maps by the autonomous driving industry in early 2023, the approach of various leading companies have largely converged to focus on the perception component of large models: Transformer-based bird’s eye view (BEV), Occupancy, and road network topology.
Following this trajectory, they are gearing up to prioritize the development of planning and control modules. These will foreseeably be integrated into a neural network, with the eventual aim of achieving end-to-end autonomy.
For instance, Huawei’s recent advanced driving system (ADS) 3.0 introduced a large model that prioritizes perception. Xpeng also introduced a neural network-based large model called XPlanner that focuses on planning and control, which will operate downstream of its perception-based large model.
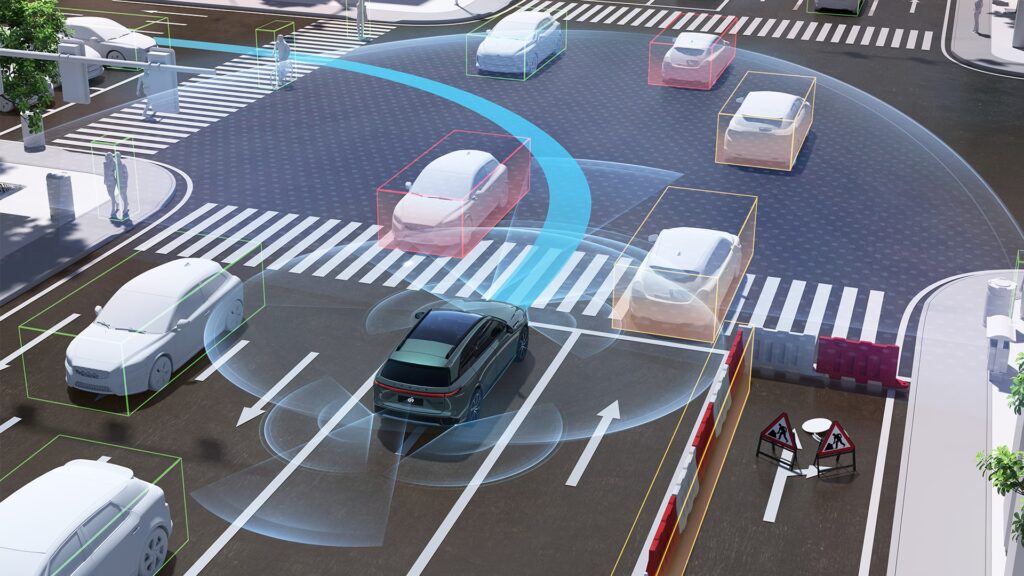
However, domestically developed solutions that are supposedly end-to-end typically still need to cycle through the perception, prediction, and planning stages, without directly outputting control commands.
Additionally, going end-to-end does not necessarily entail the complete abandonment of traditional rule-based approaches.
A technical manager from a leading automaker’s autonomous driving team explained to 36Kr that, while machine perception capabilities of large models are capable of detecting the position and color of traffic lights, some rules are still needed to guide the vehicle in scenarios where traffic lights are present in all directions.
Moreover, in terms of prediction and planning, neural networks only serve as a point of reference, with final solutions still reliant on rules.
Wu Xinzhou, the former head of autonomous driving at Xpeng and current head of Nvidia’s automotive division, said that going end-to-end represents the ultimate step for autonomous driving. However, Wu cautioned against interpreting what end-to-end represents literally, as there may be other elements involved. He stressed the need for guardrails before launching a truly end-to-end system, as continuous optimization and growth are imperative.
In essence, a blend of traditional and end-to-end approaches is likely to become the industry’s mainstream solution, with Chinese autonomous driving players expected to continuously validate them over the next 1–2 years.
Crossing the threshold
While Tesla’s FSD v12 showcases noteworthy performance, its success is not solely attributed to end-to-end solutions but also to the accumulation of millions of vehicle data points and the utilization of extensive training computing power exceeding 10 exaflops. This advantage has propelled Tesla ahead of competitors and expedited the commercialization of autonomous driving in North America.
Elon Musk’s announcement on social media regarding Tesla’s USD 10 billion investment in AI training and inference this year underscores the critical importance of financial commitment to automotive AI. Musk’s assertion that companies unable to match or efficiently utilize such spending cannot compete sets a high bar for competitors.
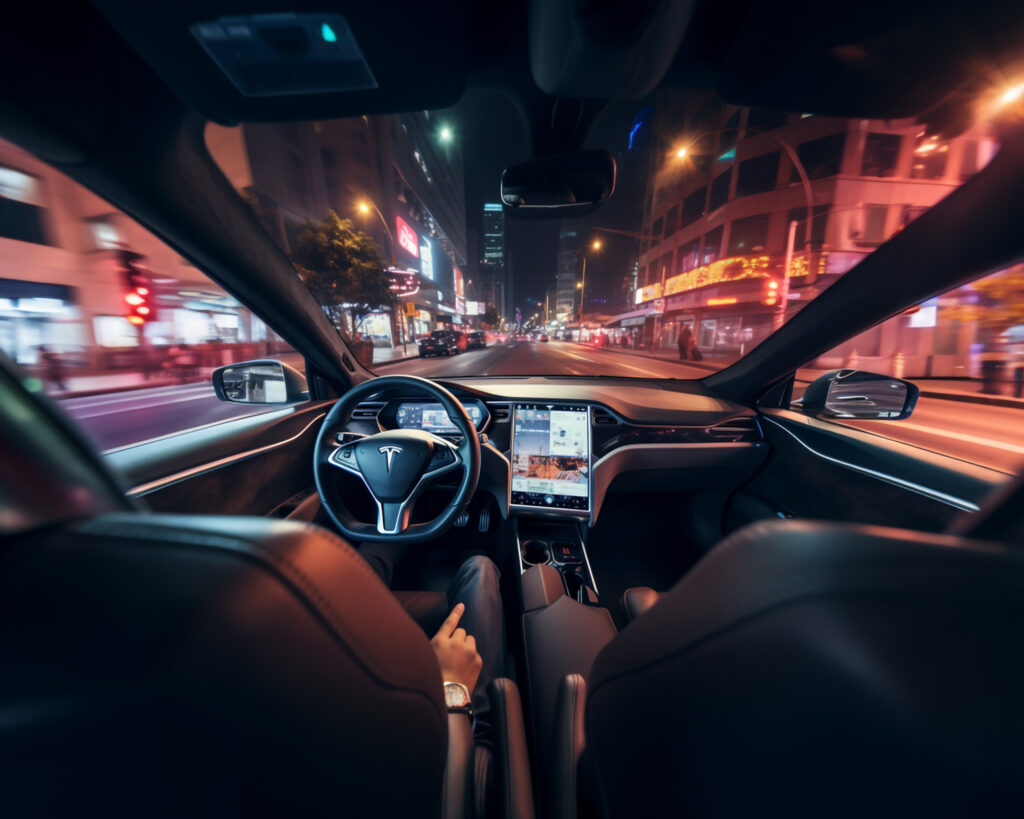
To keep pace with Tesla, players must invest not only in developing end-to-end solutions but also in accumulating data and computing power. However, the challenge lies in the dependence on high-end autonomous driving-equipped vehicle sales to accumulate substantial data, necessitating increased vehicle sales to broaden the geographical scope of data collection.
In terms of cloud computing power, Huawei currently leads the Chinese autonomous driving market, with plans to increase computer power for ADS training to 3.5 exaflops by June. Other key players, including Xpeng, Nio, and Li Auto, possess computing powers of 0.6, 1.4, and 1.4 exaflops, respectively.
Regarding onboard computing power, feedback from the industry indicates that Nvidia’s Orin chip is sufficient to support end-to-end autonomous driving solutions. Nonetheless, anticipation surrounds Nvidia’s upcoming next-generation autonomous driving chip platform, Thor, which is tailored for frameworks like Transformer and expected to boast AI computing power of 1,000 TOPS.
There has been speculation among industry insiders that Thor’s implementation will further facilitate the deployment of large models onboard vehicles, including multimodal large models, potentially transforming vehicles into more dynamic entities. Several major players, including BYD, Nio, Xpeng, Li Auto, and GAC Motor, are set to adopt Nvidia’s Thor chip, with mass production anticipated to commence in 2025.
The penetration of autonomous driving in urban areas also marks the inception of partial Level 3 self-driving capabilities in the industry.
This transition represents not only a technological and engineering challenge but also necessitates protracted adjustments in business forms, insurance, and policies. Nio’s Ren told 36Kr that he anticipates the industry will explore Level 3 solutions before pushing for widespread adoption, thereby positioning competition at the Level 3 stage as foundational for future success.
This article was written by Li Anqi in Chinese and was originally published by 36Kr
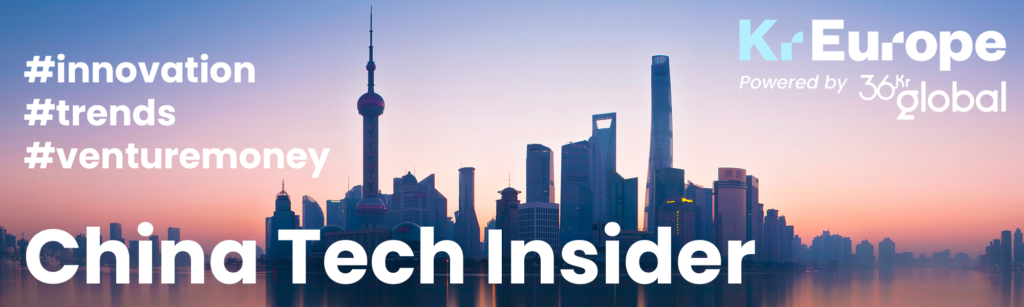