In 2024, several artificial intelligence companies in China, once ambitious about becoming the country’s answer to OpenAI, are facing a stark reality check.
According to 36Kr, two of the six Chinese AI unicorns—referred to as the six “AI tigers”—have shifted their focus away from pre-trained models. These two companies have scaled back their pre-training algorithm teams and redirected efforts toward AI applications. The media outlet did not disclose the names of the two companies, but the six AI tigers include Zhipu AI, 01.AI, MiniMax, Baichuan Intelligence, Moonshot AI, and Stepfun.
In September, speculation arose that Baidu, an early player in the large AI model space, might abandon its development of general-purpose foundational models to prioritize applications. While Baidu later denied these claims, the idea of stepping back from pre-trained models made waves throughout the industry.
Pre-training has long been the key differentiator in the AI model race, providing large models with a vast store of general knowledge. This foundational phase helps determine the performance of AI models and is often where companies derive their most significant technical advantages.
However, as third-party pre-trained models improve, many companies contemplate abandoning their own pre-training efforts in favor of post-training—customizing models to better understand specific user needs. This approach is generally more cost-effective, given the high computational power required for pre-training.
In August 2024, Character.AI, a well-known AI company from Silicon Valley recognized for its role-playing applications, announced it was moving away from developing its own pre-trained models and would instead collaborate with third-party models. According to the company’s blog, this shift would allow it to focus on post-training and enhancing the user experience for its growing audience.
For companies racing to achieve artificial general intelligence (AGI), stepping away from pre-training means effectively exiting the competition. Developing proprietary pre-trained models had secured these companies significant funding, top-tier talent, and industry recognition in a short span. By leaving pre-training behind, they risk deflating the AI technology bubble.
“Many companies have taken not OpenAI’s technology but its blind confidence in pursuing a similar path,” an AI practitioner told 36Kr.
That said, abandoning pre-trained models isn’t necessarily a bad omen for the AI sector. In an environment where funding is tight and computational resources are limited, some AI model companies are reassessing their strengths and resources. Shifting focus from models to applications suggests that many AI companies are choosing to prioritize survival before pursuing AGI.
Choosing between models and products
Scaling law—the dominant approach to pre-training—requires scaling up parameters, which in turn demands vast, ongoing investments in computational power and data. Elon Musk has estimated that training GPT-5 could require 30,000–50,000 H100 GPUs, with chip costs alone exceeding USD 700 million, roughly equivalent to Baidu’s quarterly net profit.
For startups yet to turn a profit, raising the capital necessary to scale their models remains a significant challenge. As 36Kr reported, Chinese AI unicorns now boast valuations of around RMB 20 billion (USD 2.8 billion), with recent funding rounds averaging RMB 5 billion (USD 700 million). Yet, these skyrocketing valuations have also made it more difficult to secure new funding.
One investor told 36Kr that AI unicorns developing large models are unlikely to aggressively pursue financing for the remainder of 2024. “Both the companies and the primary market are pessimistic about the next round of fundraising.”
These funding challenges are compounded by the substantial marketing costs associated with AI applications, which have struggled to achieve profitability.
Despite significant investment, the performance gap between Chinese AI companies and OpenAI remains wide, with little to distinguish the performance of models developed in China. Many Chinese firms are now focusing on AI applications, hoping to become the WeChat or TikTok of the AI era. Even traditionally B2B companies like Zhipu and Baichuan have launched consumer-facing apps, such as Zhipu Qingyan and Baichuan’s Baixiaoying.
Large-scale AI applications are increasingly viewed as the moat that keeps AI companies in the game, making user data an essential asset. Moonshot AI, for example, reportedly spent approximately RMB 30 per user on Chinese video platform Bilibili for customer acquisition, while ByteDance’s Doubao had a cost per acquisition (CPA) nearly double that.
Since early 2024, aggressive marketing campaigns have led AI companies to double or even triple their marketing budgets. While the cost of marketing continues to rise, customer acquisition remains one of the few reliable methods of gaining users in a market where AI products still lack significant differentiation.
Not all companies are optimistic about the sustainability of this approach. Although the cost of inference has dropped by nearly 99% compared to a year ago, training still accounts for at least 70% of overall computational expenses. As a result, abandoning pre-trained models is increasingly seen as the most cost-effective decision in a resource-constrained environment.
One unicorn that has exited pre-training is now focused on overseas AI applications and plans to go public in mid-2024.
The monetization dilemma
Why are AI companies shifting their focus from models to products? At its core, the issue is that there’s currently no clear path to monetizing large models.
Multiple industry insiders told 36Kr that the wave of price cuts on models in 2024 has not translated into profitability. “Model API price cuts were intended to attract customers and convert them to higher-margin services like on-premise deployment,” explained an account manager from an AI firm. “But the results have been disappointing. Most AI companies saw their B2B revenue slashed by half in the first half of this year.”
He added that when one model offered free access, a surge of developers flooded in, with a single research-focused user consuming 60% of the available tokens in just one day.
Open-source models have further complicated the revenue picture. With the release of models like Llama 3.1, Mistral Large 2, and DeepSeek V2.5, open-source performance is now comparable to, or even exceeds, that of proprietary models such as GPT-4 and GPT-4o. As a result, many companies that previously paid for model access are now building their own solutions using open-source technology.
This shift has undermined the value proposition of proprietary models. “Companies with high budgets typically have their own tech teams and can develop directly using open-source models,” the account manager said. “For companies lacking technical capability, open-source models have reshaped their expectations of what models should cost.”
In 2023, a notable example emerged with the release of Llama 2, when one AI unicorn saw a client reduce its offer to just one-tenth of the original price. The limited ability of models to generate revenue means that, for now, AI companies must rely heavily on financing or AI applications that can quickly achieve product-market fit.
Currently, only two factors are attracting investment for AI model companies: impressive user data or a significant leap in model performance. One investor told 36Kr that companies need to match the capabilities of OpenAI’s latest model, o1, if they hope to excite the primary market.
Yet, by 2024, many AI companies have hit a technological bottleneck. Following the release of GPT-4, the pace of large-model development has slowed, and multimodal AI remains in its early, challenging stages. “Before GPT-4, OpenAI published detailed technical reports, offering a blueprint for others to follow. But once OpenAI stopped releasing those reports, Chinese companies lost their guide,” an industry insider explained. “And even OpenAI’s approach may not be the right one.”
A growing number of large model developers are losing direction and seeking stability amidst technological uncertainty. Companies that have stepped away from pre-trained models are now focusing on AI applications with clearer revenue potential. Several sources told 36Kr that one company’s overseas AI productivity tool generated the bulk of its revenue in 2024. “70% of the company’s workforce is now focused on products,” and the underlying model for this tool is gradually shifting from self-developed to GPT-4 and GPT-4o.
Another company that initially focused on B2B in China also began releasing consumer-facing AI productivity and entertainment apps in mid-2024.
Meanwhile, those still committed to pre-trained models are exploring ways to cut costs and improve efficiency. One employee at an AI unicorn told 36Kr that the company has been cautious with its computational power spending, instead focusing on optimizing its training framework to reduce expenses.
For example, OpenAI’s latest model, o1, employs a self-play strategy, which enhances performance without increasing the number of parameters. This strategy has become a lifeline for companies seeking more cost-effective model training.
For the broader AI industry, abandoning pre-trained models is not necessarily a negative sign. Guangmi Li, CEO of Shixiang Tech, recently predicted that 80% of companies will abandon pre-training in the future.
The emerging consensus in Silicon Valley is that reinforcement learning could be the next major breakthrough, offering a way to fine-tune model parameters while controlling costs.
This shift indicates that, after a period of hype, companies are returning to rational thinking and reassessing both their technological paradigms and resource allocation strategies.
This article was written by Zhou Xinyu and was originally published by 36Kr.
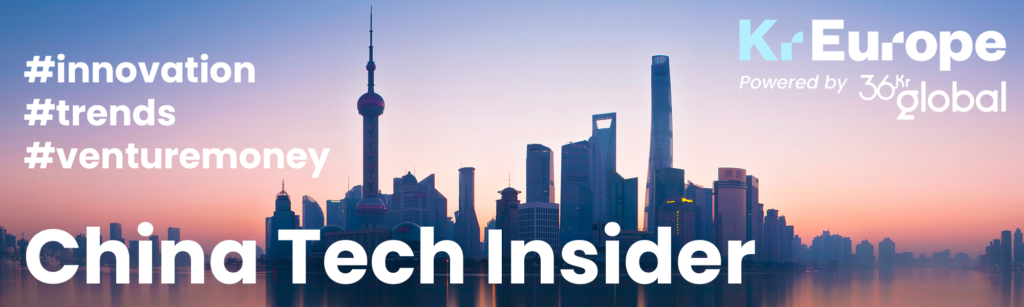